Customer-centricity and financial inclusion remain a challenge for the financial services sector. With banking products offered to customers becoming increasingly commoditized, AI-powered analytics can help banks differentiate themselves, provide competitive edge and enable personalised customer experience. The coming of age of such technologies allows banks to develop a strategic and organizational focus in analytics and adopt it as a true business discipline.
by Krish Narayanaswami, President & Global Head – Banking at Azentio Software
The analytics and business intelligence (BI) software market grew by 10.4% to $24.8bn in 2019. The world of banking has encountered unprecedented change over the past few years, and trends show that this will be the norm for a while longer. Modern BI platforms continue to be the fastest-growing segment at 17.9%, followed by data science platforms with a 17.5% growth (Source: Gartner).
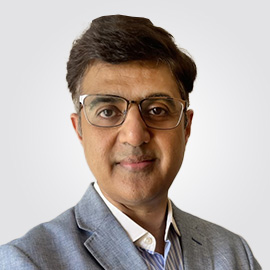
Banks have been an early adopter of BI and analytics to drive business growth, reduce risk and optimise cost. With the rapid growth in digital banking, the velocity of transaction data has increased multifold. This data has now unlocked tremendous opportunities for banks in understanding consumer behaviour and tailor make specialised offerings by leveraging analytics as Decision as a Service
How will changes in banking laws and regulations affect profitability? Which stress scenarios should be considered? Who are the current ‘high-value’ customers? Which customers have the highest potential to ensure revenue growth? Can the bank create an early warning system to prevent fraud by identifying patterns? Increasingly, data analytics is seen as the answer that banking leaders are looking to, to successfully navigate this volatile environment.
Present Day Strategy and Key Drivers
According to a recent Deloitte survey, frontrunners benefited from early recognition of the importance of analytics to the overall business success. This recognition has helped them shape a specific analytics implementation plan that considers holistic AI adoption across the enterprise. The survey indicates that many frontrunners launched analytics centres of excellence and established comprehensive, companywide strategies for AI adoption for their internal departments, recognizing the strategic importance of AI.
The following strategies should be taken into consideration while adopting an analytics framework across the organization.
Prioritize the focus areas
Identify key areas where data and analytics can have the greatest impact and obtain leadership engagement from the start (for example, customer, risk, finance). This must reflect in the immediate goals and vision of financial institutions.
Streamline your data
Provide an integrated view of high-quality data vs. siloed pockets across product and business lines (for example, single view of the customer, aggregated risk exposure by product). Setting up a data warehouse/data lake and further creating specialized data marts to make the data more structured and easily accessible to the respective stakeholders.
Integrate with decision management systems
The key is to develop data-driven strategies at every step to arrive at smart decisions. Analytical insights can be plugged directly into decision management systems to arrive at the next best action.
Onboarding the right skillsets
Finding the right talent for statistical modelling little data and big data is one of the biggest challenges. Develop a talent plan that builds on both existing internal talent and external sources.
Leverage the power of cloud
Leading cloud providers like AWS, Azure, OCI and GCP are providing powerful analytical offerings as services and have the power to run heavy compute. The ability to scale up infrastructure to run high loads and to bring it down when not required helps in optimising costs.
What is the way forward?
According to a 2020 McKinsey study involving over 25 use cases, AI technologies can help boost revenues through increased personalization of services to customers (and employees); lower costs through efficiencies generated by higher automation, reduce errors rates and improve resource utilization. It can uncover new and previously unrealized opportunities based on an improved ability to process and generate insights from vast troves of data.
The potential value creation for banks is one of the largest across industries, as AI can potentially unlock approximately $1 trillion of incremental value for them annually.
Realising the need for going mainstream with AI, banks internationally have already started harnessing the power of data to derive utility across various spheres of their functioning, including sentiment analysis, product cross-selling, regulatory compliances management, reputational risk management, and financial crime management.
AI and analytics will eventually become a part of every major initiative, in areas ranging from customers and risk, to finance, workforce, and supply chain.
Personalised experiences and products powered by advanced analytics and machine learning will be key to wooing customers in this era of intense competition. Banks have a chance to overcome the hurdles and join the analytics arms race before the frontrunners extend the gap that would be too far to bridge.