Unlocking a digital future: how the finance industry can improve data quality
Deloitte’s recent predictions for the future of finance highlight the need for the finance industry to adopt the technology available to them in order to remain competitive. But for the finance sector to become truly digital, the quality of data is paramount.
By Baiju Panicker, Global CTO and Practice Head – Banking, Insurance and Financial Services at Altimetrik
Shifting to a truly digital mindset means adopting a digital business methodology that uses data to support and improve operations. However, if the data is low in quality, incomplete, or corrupted, this will make it near-impossible for the business to operate in an efficient and effective way.
Data quality critical in finance
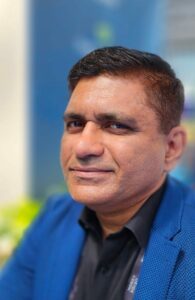
Low-quality or incomplete data can lead to poor lending, high-risk, flawed valuations and suboptimal trading. Ineffective targeting can also result from poor data, as can complaints, failures, and distorted insights.
In stark comparison, accurate data enables sound business decisions. High-quality data provides insight for analytics and efficient banking activities. It establishes greater integrity across operational analytics, fundamental to successful financial decisions and the overall success of the financial industry.
A great example of this is artificial intelligence (AI). AI is only as good as the data it accesses. It is crucial for financial firms to invest in data quality at the outset to enable successful digitisation, which in turn can boost competitiveness in the market and increase customer satisfaction.
Technology adoption critical
The adoption of technology is central to improving data quality. Leveraging various technologies to enhance data quality, such as automation tools for validation, AI for anomalies, and streaming analytics for real-time monitoring can ensure that only accurate and validated data is captured, improving the data quality immediately.
Data machine learning, blockchain, and natural language processing can help financial institutions improve their data quality and overall market performance by spotting inconsistencies, securing transactions, and extracting insights.
Without these building blocks, there is great potential for failure. Multiple client records can cause confusion, incorrect bills can damage trust, and customers and contracts may be lost.
Cleansing existing data is vital, but it is important to recognise that this cannot effectively be undertaken as a one-off project. Instead, it needs to be implemented as an ongoing activity to ensure overall business success. Alongside this ongoing process, businesses need to properly validate data as it comes in, such as automating data input and real-time monitoring to maintain a high standard of data throughout.
Utilising data stewards to monitor and address data quality issues gives a direct responsibility within the business to monitor data, clearly setting out the business’ intention to staff, customers and stakeholders that data quality is at the heart of the organisation and its operations.
Building a sustainable data quality framework
Undoubtedly, there will be lots of challenges that businesses face whilst undertaking this process. Focusing on a short-term goal – such as a single data cleanse – can be short-sighted and only create the same problems further down the line. Ensuring coordination across the business is key to success, which leads to greater accountability and removes silos from the process.
Machine learning and rule-based detection can support teams and help avoid any deviation from the prescribed style of data being captured. Text mining and natural language processing can help businesses analyse documents, call transcripts, and social media posts to identify semantic anomalies and outliers that indicate data quality issues. Alerts can then be set up to flag when issues emerge.
Ultimately, combining technology-driven detection with business-driven strategies for ongoing data quality improvement will enable businesses to be vigilant regarding poor quality or erroneous data being captured and utilised.
How to ensure quality
Proactive checking of data for errors and maintaining its quality is vital to the whole process of data quality, as early identification of problems helps to establish trust. Establishing a governance structure internally, where all parties are aware of and active in their roles, is fundamental both from a business perspective and also for stakeholders and customers.
Cross-functional data governance is important. It is not enough for each department to run its own checks and processes; it needs to be business-wide, with no silos or breaks in communication. This is where a Single Source of Truth (SSOT) is important. Rather than having multiple data locations that might not interact with other departments or processes, holding all the information centrally allows for better data accuracy and effective data cleansing across the whole business.
Overarching benefits of high-quality data
The potential benefits of improved quality of data to financial organisations are manifold. There is huge potential for increased revenue and cost savings through optimised data-driven decisions and operations. Data-driven activity is always more accurate, and data quality is central to this. The results are improved customer satisfaction and retention, with improved product offerings based on accurate findings.
From an operational perspective, management will see higher employee productivity with reliable data to work with, coupled with higher staff satisfaction. Through the integration of accurate, high-quality data there can be an increased use of automation and AI for more mundane tasks, enabling employees to work on more challenging and rewarding activity.
The finance industry is at a crucial juncture when it comes to digital adoption. Those who embrace digital adoption and intelligent ways of working through data and intelligent analytics will thrive, whilst those who lag behind will struggle to compete against competitors with a digital business mindset.