Can ChatGPT help fight cybercrime?
Open AI’s ChatGPT has taken the world by storm, with its sophisticated Large Language Model offering seemingly endless possibilities. People have put it to work in hugely creative ways, from the harmless scripting of standup comedy to less benign use cases, from AI-generated essays that pass university-level examinations to copy that assists the spread of misinformation.
Iain Swaine, Head of Cyber Strategy EMEA at BioCatch
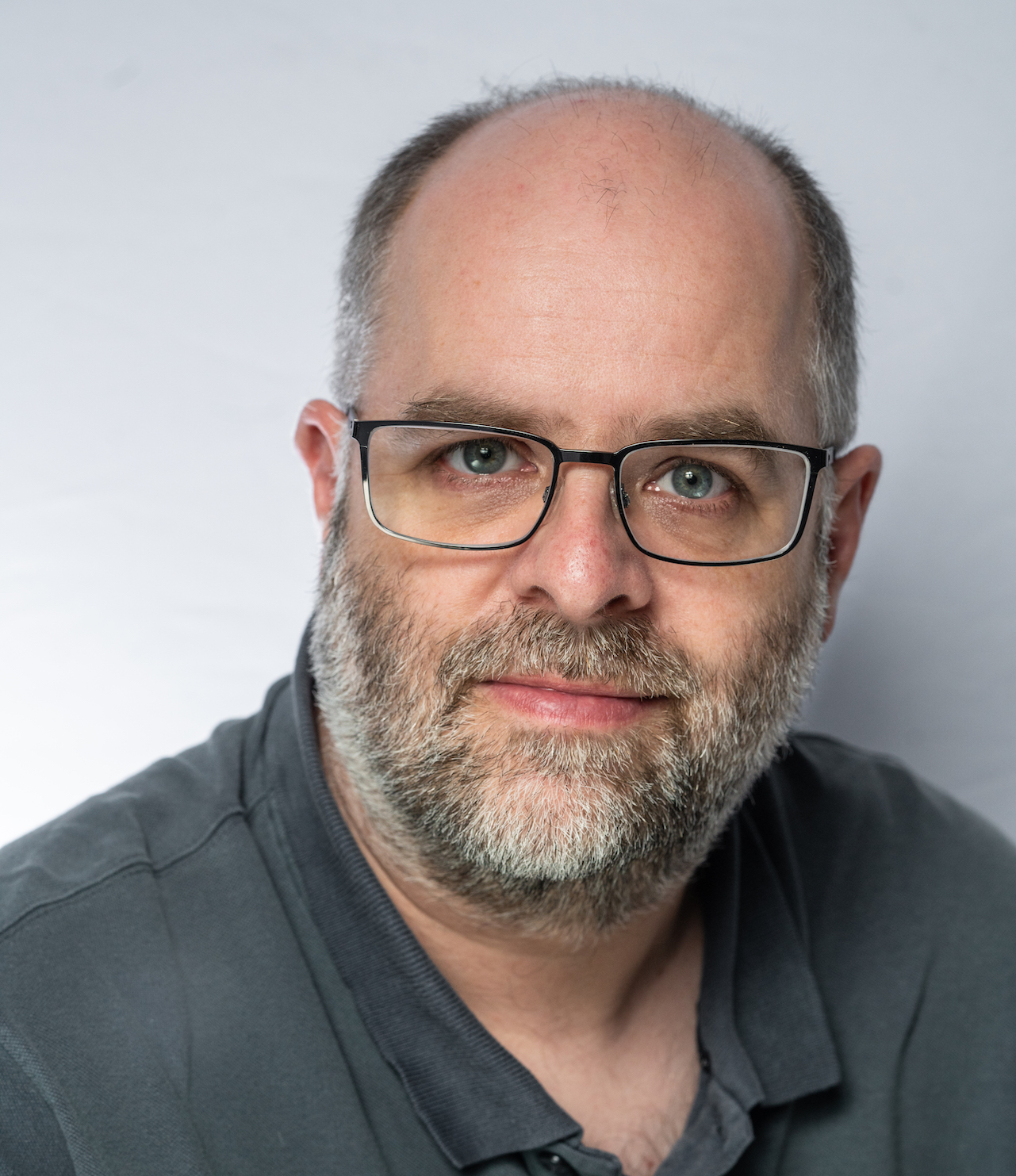
Chat GPTs (Generative Pretrained Transformers) are a deep learning algorithm that generates text conversations. While many organisations are exploring how such generative AI can assist in tasks such as marketing communications or customer service chatbots, others are increasingly questioning its appropriateness. For example, JP Morgan has recently restricted its employees’ use of ChatGPT over accuracy concerns and fears it could compromise data protection and security.
As with all new technologies, essential questions are being raised, not least its potential to enable fraud, as well as the power it may have to fight back as a fraud prevention tool. Just as brands may use this next-gen technology to automate human-like communication with customers, cybercriminals can adopt it as a formidable tool for streamlining convincing frauds. Researchers recently discovered hackers are even using ChatGPT to generate malware code.
From malware attacks to phishing scams, chatbots could power a new wave of scams, hacks and identity thefts. Gone are the days of poorly written phishing emails. Now automated conversational technologies can be trained to mimic individual speech patterns and even imitate writing style. As such, criminals can use these algorithms to create conversations that appear to be legitimate but which mask fraud or money laundering activities.
Whether sending convincing phishing emails or seeking to impersonate a user and gain access to their accounts or access sensitive information, fraudsters have been quick to capitalise on conversational AI. A criminal could use a GPT to generate conversations that appear to be discussing legitimate business activities but which are intended to conceal the transfer of funds. As a result, it is more difficult for financial institutions and other entities to detect patterns of money laundering activities when they are hidden in a conversation generated by a GPT.
Using GPT to fight back against fraud
But it is not all bad news. Firstly, ChatGPT is designed to prevent misuse by bad actors through several security measures, including data encryption, authentication, authorisation, and access control. Additionally, ChatGPT uses machine-learning algorithms to detect and block malicious activity. The system also has built-in safeguards against malicious bots, making it much harder for bad actors to use it for nefarious purposes.
In fact, technologies such as ChatGPT can actively help fight back against fraud.
Take Business email compromise fraud (BEC). Here a cybercriminal compromises a legitimate business email account, often through social engineering or phishing, and uses it to conduct unauthorised financial transactions or to gain access to confidential information. It is often used to target companies with large sums of money and can involve the theft of funds, sensitive data, or both. It can also be used to impersonate a trusted business partner and solicit payments or sensitive information.
As a natural language processing (NLP) tool, ChatGPT can analyse emails for suspicious language patterns and identify anomalies that may signal fraud. For example, it can compare email text to past communications sent by the same user to determine if the language used is consistent. While GPT will form an essential part of anti-fraud measures, it will be a small part of a much bigger toolbox.
New technologies such as GPT mean that financial institutions will have to strengthen fraud detection and prevention systems and utilise biometrics and other advanced authentication methods to verify the identity of customers and reduce the risk of fraud. For example, financial organisations already use powerful behavioural intelligence analysis technologies to analyse digital behaviour to distinguish between genuine users and criminals.
In a post-ChatGPT world, behavioural intelligence will continue to play a vital role in detecting fraud. By analysing user behaviour, such as typing speed, keystrokes, mouse movements, and other digital behaviours, behavioural intelligence will aid in spotting anomalies. These can indicate that activities are not generated or controlled by a real human. It is already very successfully being used to spot robotic activities which are a combination of scripted behaviour and human controllers.
For example, a system can detect if a different user is attempting to use the same account or if someone is attempting to use a stolen account. Behavioural intelligence can also be used to detect suspicious activity, such as abnormally high or low usage or sudden changes in a user’s behaviour.
As such, using ChatGPT as a weapon against fraud could be seen as an extension of these strategies but not as a replacement. To counter increasingly sophisticated scams, financial service providers such as banks will need to invest in additional control such as robust analytics to provide insights into user interactions, conversations, and customer preferences and comprehensive audit and logging systems to track user activity and detect any potential abuse or fraudulent activity.
And it’s not all about fraud prevention. Financial institutions should also consider how they use biometric and conversational AI technologies to enhance customer interactions. Such AI-driven customer service platforms can ensure rapid response times and accurate resolutions, with automated customer support services providing quick resolutions and answers to customer queries.
Few world-changing technologies arrive without controversy, and ChatGPT has undoubtedly followed suit. While it may open some doors to criminal enterprise, it can also be used to thwart them. There’s no putting it back in the box. Instead, financial institutions must embrace the full armoury of defences available to them in the fight against fraud.